The deputy head of NATO’s Innovation Unit lays out current efforts to develop Artificial Intelligence policy at NATO.
As noted in the first article in this series on innovation at NATO, the Alliance faces a global technology adoption race. Rival powers are leveraging new technologies to pursue the dual goal of greater economic competitiveness alongside greater military capabilities. The Allies face a range of challenges as they seek to exploit emerging and disruptive technologies. These challenges are based on two interrelated pillars of work: ensuring a dynamic adoption of new technologies and governing them responsibly. Artificial Intelligence (AI) is at the heart of these considerations.
AI is the ability of machines to perform tasks that typically require human intelligence – for example, recognising patterns, learning from experience, drawing conclusions, making predictions, or taking action – whether digitally or as the smart software behind autonomous physical systems (see Science & Technology Trends 2020-2040 – Exploring the S&T Edge).
As proposed by the RAND think tank in the report The Department of Defense Posture for Artificial Intelligence: Assessment and Recommendations (2019), it is useful to distinguish between three broad types of applications: Enterprise AI, Mission Support AI, and Operational AI.
Enterprise AI includes applications such as AI-enabled financial or personnel management systems, which are deployed in tightly controlled environments, where the implications of technical failures are low (in terms of immediate danger and potential lethality).
Operational AI, by contrast, can be deployed in missions and operations, i.e. in considerably less controlled environments and such that the implications of failure may be critically high. Examples include the control software of stationary systems or those of unmanned vehicles.
Mission Support AI, an intermediate category in terms of environment control and failure implications, includes a diverse set of applications, e.g. logistics and maintenance, or intelligence-related applications.
These distinctions may prove useful in setting priorities, both for adoption policies and for principles of responsible use, taking into account the differing levels of risk inherent to these categories.
The contemporary wave of AI, or Second Wave AI, is centered on Machine Learning (ML). ML involves the development and use of statistical algorithms to find patterns in data. For example, a classification algorithm can be trained on a large set of correctly labelled examples to determine to which previously encountered category a newly observed object belongs. Deep Learning is a subset of ML, which uses multiple computational layers (Artificial Neural Networks with multiple layers) for the handling of computationally demanding pattern recognition or prediction problems, e.g. Convolutional Neural Networks for object detection within images. Figure one below is an illustration of Deep Learning for the identification of planes, vehicles and buildings within images.
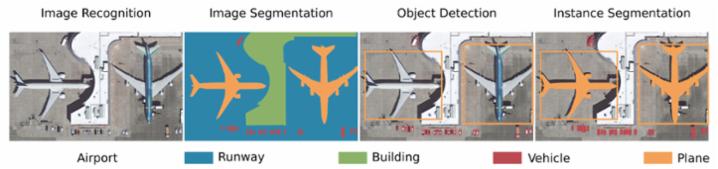
Deep Learning can be used to detect specific objects within images.
(Source: Hoeser, T.; Kuenzer, C. Object Detection and Image Segmentation with Deep Learning on Earth Observation Data: A Review-Part I: Evolution and Recent Trends. Remote Sens. 2020, 12, 1667.)
ML thrives on large sets of accurate data and performs poorly with small datasets or with inaccurate data. Under good conditions across a wide range of uses, ML outperforms humans, in terms of both predictive performance and of course speed, over an increasing range of narrow pattern recognition and prediction tasks. This is the central reason for the increasing adoption of ML across vast areas of human activity.
Towards dynamic adoption
Traditionally, economists have modelled output as a function of labour and capital (production factors), and material inputs. For AI, the production factors are high-skill specialist talent and Information and Communication Technologies (ICT) infrastructure for computing and storage, and data is the key input.
Is data then the new oil? Essentially no. While data does need to be ‘extracted’ and then ‘refined’ before further use, its availability grows with the volume of output. Data is also specific, not fungible. For any specific use case, specific datasets that capture exactly relevant real-life observations (or simulations) are needed. To ensure a solid starting point, Allied defence establishments will need to ensure their further digitisation. Overall, data policy needs to address a full value chain, covering collection, access, sharing, storage, metadata, documentation, quality control (including data cleaning and bias mitigation), and processes to ensure compliance with legal requirements.
Supporting measures for the production factors – talent and infrastructure – should include sound and flexible human resources and contracting policies to attract and nurture the best human talent, as well as the deployment of relevant and secure computational and data storage capacities.
Next is productivity. Through the ages, economic actors have sought the best ways to combine the factors of production: Are they complementary? Are they substitutable? What combinations bring the highest returns? What production and managerial processes and practices yield the best outcomes? For AI, while trade-offs exist to some extent, the factors of production are far more complements than they are substitutes: one needs substantial investments in all three factors to succeed.
As for processes and management, software industry best practice indicates that the development and delivery of AI solutions should rely on Agile approaches, rather than on a traditional Waterfall model. This implies highly dynamic and iterative processes, starting from an initial and flexible problem statement, rather than a detailed and rigid statement of requirements. Several variants of Agile exist in industry and some of these variants are actively pursued within certain NATO communities.
Committing to responsible use
The Alliance’s success with AI will also depend on new and well-designed principles and practices relating to good governance and responsible use. Certain Allied governments have already made certain public commitments in the area of responsible use, addressing concepts such as lawfulness, responsibility, reliability, and governability, among others.
In parallel, Allies have taken part in the Group of Governmental Experts on Lethal Autonomous Weapon Systems under the auspices of the United Nations, leading to the formulation of 11 guiding principles.
Importantly, there is a good case for viewing work on adopting AI and work on principles of responsible use as complementary and synergistic. In effect, there are certain essential principles or goals that will underpin and facilitate both engineering good practice, as well as responsible state behaviour.
Certain national principles imply a need for specific design requirements. For example, a principle of governability may be linked to technical abilities to detect and avoid unintended consequences, and to disengage or deactivate in case of unintended behaviour.
The technical characteristics required to ensure that these and other objectives are met will necessarily be part of the design and testing phases of relevant systems. In turn, the relevant engineering work will be an opportunity to refine understanding, leading to more granular and more mature principles. Further work in the area of Testing, Evaluating, Verifying and Validating (TEVV) will be essential, as will support from relevant Modelling and Simulation efforts. NATO’s well-established strengths in the area of standardization will help frame these lines of effort, while also ensuring interoperability between Allied forces.
In the meantime, overarching principles such as those developed in some national cases, as well as under UN auspices, offer a baseline for further consultations among Allies, as well as points of reference concerning existing national positions.
This is the second article of a mini-series by NATO’s innovation team, which focuses on technologies Allies are looking to adopt and the opportunities they will bring to the defence and security of the NATO Alliance.